Next-generation sequencing (NGS) emerged in the mid-90s and became commercially available in 2005. Since the beginnings of NGS, there has been a vital need for standardization of the genomic variations, particularly in interpretation and reporting.
Each individual human is priceless and unique, and so is their genetic information. NGS generates genetic datasets used in diagnostics, treatment, and prevention. These datasets are essential for cancer detection and therapy selection, as well as prognostics.
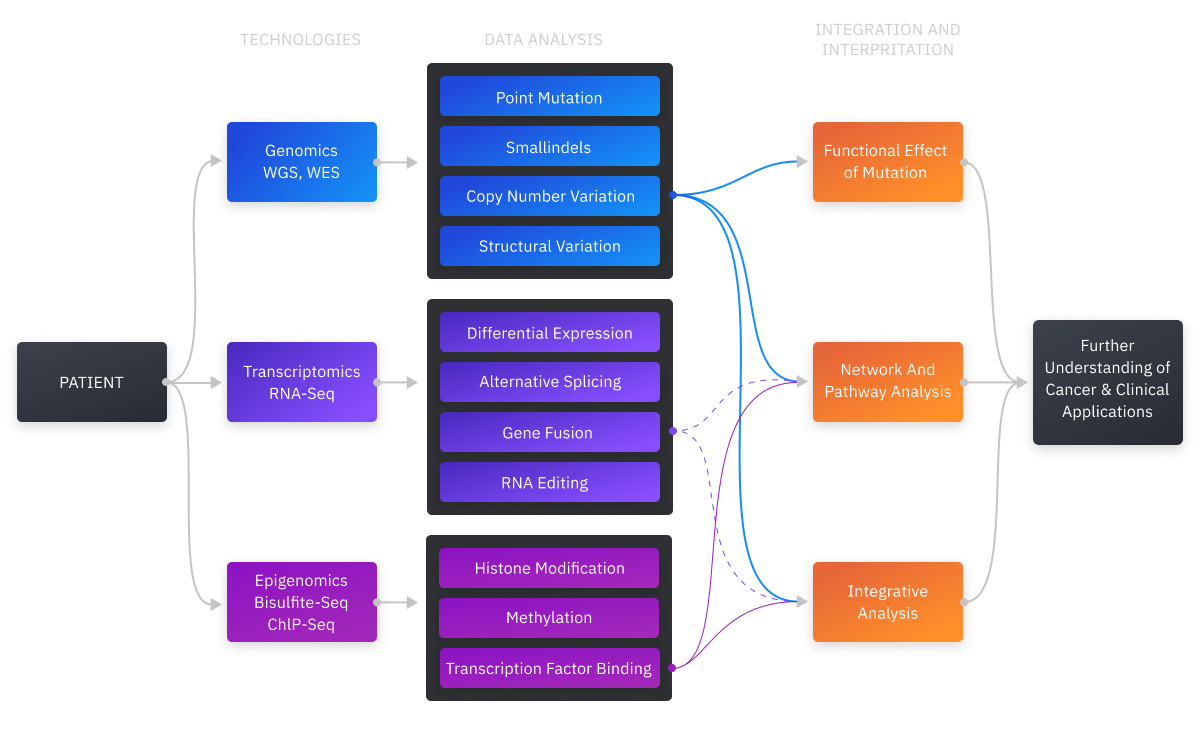
Researchers and clinicians can use next-generation sequencing to track and focus on genes that are potentially instrumental in oncogenesis. Data analytic tools and software have become cost-effective ways to get higher resolution. MarketsandMarkets estimates that the global NGS market will reach $24.4 billion by 2025, with a projected annual growth rate of 20.9%. MarketsandMarkets bases this on:
- a surge in treatment options
- application of precision medicine
- innovative next-generation tools
- reduced capital requirements for NGS solutions
The demand for genetic clinical services will continue to grow exponentially. However, the shortage of genetic clinical experts is already being felt. The gap between supply and demand will be filled by automation.
“Population research has a fundamental role to play in our vision of 3 in 4 people surviving cancer by 2034, particularly in early diagnosis and prevention. Over many years we’ve funded some of the world’s best researchers in this field. Their work has led to the introduction of screening programmes and changes in prescribing regimens and clinical practice; now—with the revolution in big data collection and processing—such studies can be better integrated into discovery and clinical research, creating a two-way flow of information.”
— Fiona Reddington; former Head of Population Research Funding; Cancer Research UK
Methods of Cancer Analysis Research
Mathematical approaches to cancer analysis make data evaluation possible, and ensures data sets are analyzed in a similar way. Cancers are heterogenous with various subtypes. In recent years, machine learning has been used to model the progression of cancers and further treatment. The major benefit of ML tools is their capacity to detect patterns and key features in immense data sets. The following techniques are applied in cancer research:
- Decision Trees (DTs)
- Support Vector Machines (SVMs)
- Artificial Neural Networks (ANNs)
- Bayesian Networks (BNs)
These techniques are used to build predictive models, which increase precision in decision-making.
High Throughput Technologies (HTTs) have produced a large amount of cancer data now readily available to the healthcare research community. Prediction accuracy is a crucial challenge for medical researchers. Machine learning techniques identify patterns and relationships between them, and use the patterns to make predictions.
“New cancer treatments are being developed and approved at a faster rate than at any time in history and our health systems are constantly evolving. Using big data to examine cancer survival and trends across populations is crucial to identify successes and highlight blockages to make sure that effective treatments are reaching all the people who need them. This research also plays a vital role in helping recognise, understand and address the long-term effects of treatments that can affect the quality of life of the growing number of cancer survivors.”
— Victoria Forster; Cancer Research Scientist, Science Communicator, and Cancer Survivor
Variant Analysis: Cancer Annotation and Filtering
Variant calling is a procedure where a given genomic sequence is compared with a reference genome and the differences are measured. Cancer bioinformatic pipelines call for variants on the basis of aligned reads generated by a sequencer. SNVs (Single Nucleotide Variants) are the most common variant calls. Variant calls are normally stored in VCF format. VCF format records an observed genotype at its specific genomic coordinate along with technical data. The determination of somatic mutations is carried out during filtering and annotation.
After the variants are called, a quality confirmation follows. The identified variants are reviewed and annotated accordingly. These annotation and filtering procedures suggest the following workflow:
- variant annotation based on gene location and changes in gene protein product
- comparing and retrieving annotations for mutations that match
- detection of poor sequencing quality variants
- somatic mutations are compared with normal tissue
- preparation of a final list of possible somatic mutations plus annotation data
Benefits of Building Clinical Variant Analysis for Cancer
“One of our biggest challenges in clinical oncology today is to figure out how to obtain ‘actionable’ molecular subtyping of every newly diagnosed cancer without spending $1 million to do so.”
— Christopher C. Benz, MD, Professor and Program Director, UCSF/Buck Institute
Over the last decade, we have conducted multiple studies and had conversations with clinicians, academics, and researchers. These dialogues have shown us the limitations and challenges of variant analysis, which are crucial for further exploration in this area. Based on our experience, the tools and software for clinical variant analysis in the context of cancerous diseases have the following mission:
- to consistently deliver high-quality interpretation
- to provide a framework for clinicians with less experience
- to be ahead of the curve in the latest developments
“Our medical research products combine cutting-edge technologies to provide two crucial things: scalability and reliability. Cancer research is on the verge of many breakthroughs. This is a time where speed, cost-effectiveness, and quality are vitally important. These are the immutable pillars of our solutions.”
— Vlad Medvedovsky, Founder and Chief Executive Officer at Proxet, custom software development solutions company.
We build software for cancer variant analysis with the following features:
- easy aggregation of data from various sources
- robust and customizable reporting system
- user-friendly and intuitive interface
- effective categorizing and filtering
- streamlined assessment
- holistic variant annotation
Early-stage cancer detection can give second chances. Proxet has experience with healthcare research institutes, clinicians, and projects of different kinds. Given the increasing pace and size of data volumes, new approaches to variant analysis will require tools that increase accuracy and reliability. In addition to designing and building the tools, software developers have to weigh the marginal cost and gains of tweaks and modifications of these tools. Including future medical costs in the cost-benefit analysis of cancer is necessary for patients who are in need of costly lifelong treatment. The absence of a pharmacoeconomic framework hinders comparability and impairs decision-making.
According to “Statistical Methods In Cancer Research” by N.E. Breslow, the case-control method of analysis has been around for six decades, but the selection of control groups has presented a serious challenge. Breslow emphasized the value of case-control studies in cancer research, but also questioned case-control’s foreseeable future and alternatives. He argued that even well-managed studies were prone to flaws, and their designs had inherent scientific problems. Today, integrative analysis of complex cancer genomics and clinical profiles can be carried out with the help of open source web portals, which contain a comprehensive database. Scientists have multiple dimensions of cancer genomics data at their disposal to explore, visualize, and analyze.
In sum, widespread implementation of next-gen sequencing created a multitude of variants that require further categorization and analysis. On one hand, each data source—and there are lots of these—contain valuable information about the extent of variant relevance. On the other hand, we can use algorithms to identify and generalize about the impact some particular variant has on a protein or gene. Manual variant analysis is no longer cost- or time-efficient, so it is time to delegate essential, demanding, and high-precision tasks to new automated solutions. Data is gold.
If you have any questions, please, feel free to contact us.