Banking operations are a vital, yet costly part of any financial institution's expenses. In 2019, McKinsey reported that middle and back-office costs accounted for 18% of banks' budgets. Being able to reduce those costs while also improving both the velocity and accuracy of those processes directly affects a bank's bottom line.
Operations also touch on regulatory and client satisfaction issues. One of the ways financial institutions are meeting these seemingly opposite goals is to automate processes. However, simply attaching a computer to a problem isn't enough, especially as the conditions around the process evolve. For that reason, the industry has been an early adopter of artificial intelligence (AI) and machine learning (ML).
Banking and AI might not seem like much of a match at first. However, the need to constantly adjust systems to aspects of the environment, ranging from fraud prevention to regulation to customer expectations, means that a platform that adapts itself as well as responds to operator input makes sense.
Let's take a look at machine learning in banking.
ML in Banking
The banking industry has traditionally been an early adopter of information technologies. In particular, making operations cheaper, finding new ways to cut fraud, and improving forecasting have driven bank owners to accept technology and innovate. Considering that the same growth in IT that has fueled banking is also largely responsible for enabling new types of fraud and money laundering, it's no wonder that AI for financial services has exploded in popularity.
The strides in popularity are reflected in spending. IDC predicted that in the Asia/Pacific region alone, Financial Services company spending on the AI market is expected to increase at a 22.1% CAGR between 2019 and 2024. That's a rise from $1.584 billion to $4.298 billion in those five years. And with Asia-Pacific accounting for 15% of the world market at the time the report was released, it's easy to see that the amount of investment into AI by banking institutions globally will continue to drive innovation. But how is the banking industry connecting finance and artificial intelligence?
Types of banking operations
Banking operations teams are involved in the actual execution of transactions created by their respective units. Executing these transactions in an efficient and legal manner is an important part of an operations team's responsibility. In this article, banking operations look at ML within different aspects of banking and the business verticals it connects with. ML can be used in banking for HR and other purposes that are found in similar-sized enterprises across different industries.
Bank operations typically include some or all of the following: processing banking activities dealing with lending and savings, investments including those with equities and debt, retail and commercial activities; documentation; analysis, including macroeconomy, clients, and transactions; and regulatory and legal concerns such as reporting and fraud prevention.
Detection relationship in data
The data generated as a result of a bank's operations can be enormous, and vary greatly. Making use of this data requires the ability to make connections between seemingly disparate pieces of information. Because banking encompasses such a wide variety of functions, the operations behind them need to utilize that data to reach a wide range of business insights.
Being able to plumb the same data for different purposes is often done best by utilizing data lakes or data warehouses, depending on the use case. Regardless of the type of data store, the data itself needs to include metatags that facilitate the access by artificial intelligence platforms as well as by human users.
While the technology behind enabling AI in banking is relatively new, the benefits are certain. Capgemini points out that the banking sector is adopting AI en masse, and that there are currently no operations models without it. The reasons for doing so may vary based on the individual bank's needs, but the overall trend is clear. Modern banking institutions must use Machine Learning in their operations.

Examples of machine learning in banking
As noted above, the variety of operations across banking functions precipitates different uses for the data at hand. However, there are some similarities despite those differences. For example, Know Your Client and Anti-Money Laundering (KYC/AML) requirements are the norm in banking operations of most types. Machine Learning can be an important tool for improving the accuracy of a bank's KYC/AML functions. Training a bank's algorithms to work with the data can be started with a prepared data set.
A 2016 LexisNexis report on technology in banking included the results of a survey related to the topic. The survey showed that 42% of the high-level bankers who responded to the poll considered ML for KYC/AML to be a tool that would be commonplace within five years. Machine learning financial services platforms were already in use in 15% of respondents' banks. Furthermore, only 9% of the bankers saw no role for machine learning in the KYC/AML role.
The 42% of the bankers who saw increasing uptake in these bank operations were correct. The LexisNexis authors point out that at the time, systems without the capacity to learn from their prior processes were becoming a barrier to streamlining bank operations. Moreover, the false positives that KYC procedures generate need to be handled manually in a non-ml environment, which raises costs. Banks with adaptive technology were able to better serve a broader and more reliably validated set of clients while lowering costs.
In a similar vein, fraud detection is something that machine learning in banking can handle very well. As with KYC/AML rules, avoiding fraud is easier if the system cannot adapt to changing attempts to commit new types of banking crimes.
The fact that IT can speed up processing by bypassing manual controls is well known. The difference in using a ML system for detecting fraud will come down to accuracy and speed when detecting anomalous transactions. Capgemini, in its 2021 report on retail banking, pointed out that the speed of 5G mobile services helps facilitate real-time fraud detection. The report's authors posit that with faster services, disruptions to the bank's systems due to the need to update security policies will be minimized. Moreover, 5G aids in the ability to utilize Multi-Factor Authentication (MFA). However, MFA relies on the use of varied information types, ranging from thumbprints to entering codes to presenting valid ID. Adaptive systems utilizing machine learning financial services platforms will be more adept at correctly handling MFA's variety.
In both the KYC and AML examples, bankers need to be able to make decisions about letting processes begin—as in setting up accounts, and complete, such as with payments. Both of these work best with an understanding of the client and of client behavior. Banking artificial intelligence is seen as a route to achieving this understanding. The LexisNexis survey showed that gaining a better understanding of the bank's clientele was the primary benefit for respondents. With better insight into clients, the bank not only protects itself, but also has a better ability to offer targeted services to those clients.
"Our experience with both machine learning and banking has shown us the importance of the banks having an understanding of their clients, but also of our knowing who the bank is. Banking is often a collision of conservative, regulation-based event management with a flexible automated and adaptive platform. Having an IT partner who knows the material, but also understands the need to listen to the client, is key to a successful banking operation."
— Vlad Medvedovsky, CEO at Proxet (ex - Rails Reactor) - a custom software development company.
Machine learning use cases in banking
The examples above are only a sample of the ways that the technology facilitates banking operations. They are by far not the only machine learning use cases in banking. Because operations span the whole of the mid-and back-office, there are a lot of different uses for AI and ML in the industry. Also, as banking evolves and incorporates new services such as the possibilities of digital currencies, as seen with China's and the EU's experiments with Central Bank Digital Currencies, the need for IT-driven operations support will only grow. Here are just a few use cases taken from today's banking industry:
Retail sales payment processing
Banking and merchandise sales have converged on AI in payments processing due to the rise of a set of technologies such as the Internet of Things, robotic process automation (RPA), and non-SQL databases. This is only part of the story, however, because seemingly unrelated technologies are also playing a part in the uptake of AI in payment processing. As mentioned previously, 5G communications technology helps with fraud prevention because of the increased speed of communications. Payment processing through the use of these technologies has also become easier and cheaper.
The orchestration of all these different services and technologies in a retail setting makes use of artificial intelligence at several points. From adaptive, self-learning communications routing to fraud detection to analyzing trends and forecasting, AI platforms streamline what would otherwise be cumbersome if not impossible.
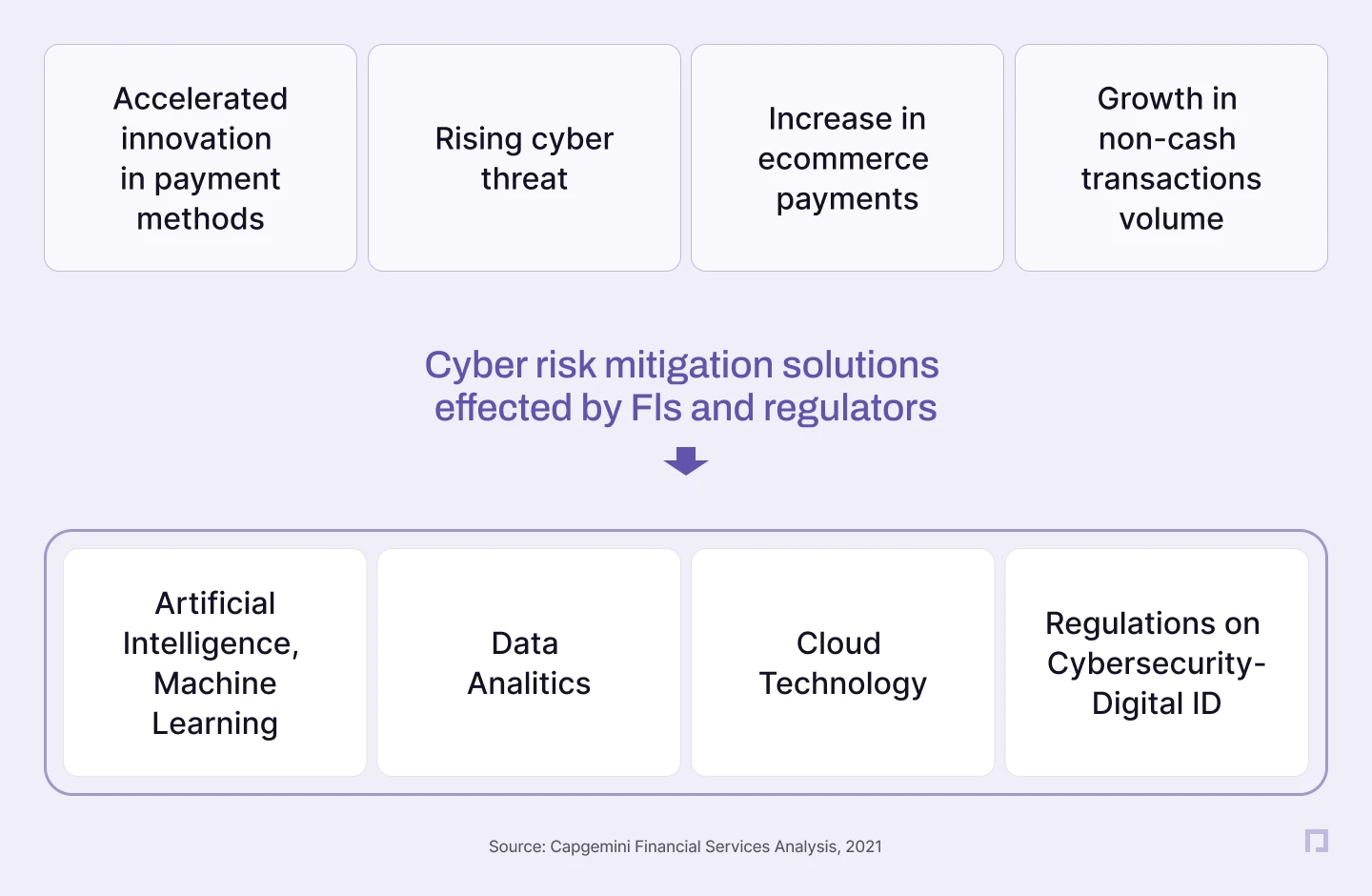
Retail banking
Retail banking, which includes banking services such as lending and savings, and cash services, is operations intensive. In countries that still use a lot of physical currency, inventories of that currency need to be maintained. While the basic patterns for cash flow through a bank may seem obvious, maintaining the availability of cash is important for maintaining confidence in the bank itself and the banking system in general.
AI can be used to better predict cash inventory needs than non-learning systems. This is because the AI platform can incorporate a wider variety of indicators and keep up with changing conditions.
Understanding those changing conditions falls under a wider scope than just cash inventory control. Forecasting interest rate changes, lending demand, and the viability of new services all benefit from AI-centered platforms. In an environment of increasing competition and rising customer expectations, greater forecasting accuracy is a must-have.
The diversity of inputs for forecasting will only continue to grow given the volatility in world markets due to factors such as public health issues and war. Changes in supply, logistics, and demand will all factor into larger pricing changes for equities and debt, which retail banks also make use of in creating investment packages for clients.
Compliance
The cost of non-compliance in the banking industry is huge. Worldwide, financial institutions paid record-breaking $10.6 billion in penalties for non-compliance. Much of this comes from violating AML rules and sanctions. Most banks have good procedures in place but fail to implement them. The human factor cannot be avoided in this case. Adaptive platforms using ML can avoid both mistakes and deliberate errors, as well as flag suspicious transactions that do go through.
The Global Association of Risk Professionals article in 2021 pointed out that improvements to compliance will take cooperation between the Chief Compliance Officer (CCO) and Chief Information Officer (CIO). Artificial intelligence and finance need to come together not only on the computer screen but also at the executive level, where the decisions—and coordination—across operations are determined. The payoff for that coordination is in more accurate compliance determinations. And because compliance and reporting cover activities across the mid-and back-offices, looking at compliance only through the lens of AML or fraud will increase the risk of costly mistakes.
Conclusion
Bringing adaptive and self-learning platforms into a financial institution's operations is the direction the industry is already heading. More streamlined and more accurate processes result, but they are dependent on cooperation starting in the C-suites. That cooperation also pays dividends as both the technology and subject matter experts are required when bringing in outside partners, especially in technology, who can facilitate platform adoption. Being able to reach out to a technology company with a focus on the banking industry and ask intelligent questions about how technology such as ML can lower costs, improve accuracy and foster innovation will prove itself in success in the marketplace.