Machine learning technology is increasingly penetrating everyday life, to the point where we do not even realize that artificial intelligence formed our feed on Instagram and other social networks. Of course, AI also handles serious tasks, such as forecasting the demand for goods, and face, fingerprint, and voice recognition.
However, it is difficult to imagine a more important and promising field of AI application than healthcare. Neural networks are expected to generate serious advances; from the diagnosis of various diseases and the development of new drugs to new treatment methods. How justified are these expectations?
As electricity changed this world and led to a new industrial revolution in the 19th century, artificial intelligence will become one of the main drivers of profound transformation of society and the economy in the 21st century.
According to IDC, the market for cognitive systems and AI technologies in 2016 was approximately $7.9 billion. Analysts believe that the average annual growth rate (CAGR) will be at least 54% by the end of this decade. Moreover, IDC believes that worldwide revenues for the artificial intelligence (AI) market will break the $500 billion mark by 2024.
The largest share of this market will be cognitive applications that automatically study data and make various estimates, recommendations, or predictions. Investments in AI software platforms that provide tools, technologies, and services based on structured and unstructured information will be at least $2.5 billion per year. According to Frost & Sullivan estimates, the market for artificial intelligence in health and life sciences will also grow at approximately 40% per year.
Thus, it is vital to consider the compatibility of machine learning and medicine in 2021.
Neural Network Benefits
The benefits of AI and neural networks can be divided into two parts: benefits to doctors and other medical providers, and benefits to patients.
Benefits of neural networks for doctors and other medical providers
Deep neural networks (DNN) can help interpret medical scans of pathologies, electrocardiograms, and endoscopy. Particular attention is paid to radiology, namely, in using neural networks to analyze X-ray images. Google used algorithms to interpret chest scans to make 14 different diagnoses, ranging from pneumonia to cardiac hypertrophy and lung collapse. DNNs can also diagnose certain types of cancer, fractures, hemorrhages, retinopathy, skin lesions, and many other diseases. Neural networks algorithms can improve the performance of dermatologists, cardiologists, ophthalmologists, and even psychotherapists by tracking the development of depression.
The thing is, most of the studies and reports still exist only as preprints. Preprints have not been published or checked by relevant reviewers. In preprints, algorithms are tested for accuracy, which is not equivalent to clinical effectiveness. Effectiveness is normally confirmed by expensive clinical trials.
Benefits of neural networks for patients
Algorithms that patients can use on their own are developed much more slowly than those used by clinicians. In 2017, the US Food and Drug Administration (FDA) approved the Smartwatch algorithm for detecting arrhythmias. And subsequently, in 2018, Apple received FDA approval for the algorithm used in the Apple Watch Series 4. The sensors on a watch can detect the user's heart rate at rest and during physical activity. If a strong deviation is detected (compared to the expected one), the user is alerted about recording an ECG through the watch. The algorithm then interprets the results.
Some smartphone apps use neural networks to monitor and control medication intake. For example, AiCure forces a patient to take a selfie video while swallowing a prescribed pill.
Algorithms can track and analyze glucose level rises or falls in patients with diabetes to prevent episodes of hypoglycemia. Thus, common chronic conditions such as hypertension, depression, and even asthma can be better controlled with the apps' help.
Practical Application of Artificial Neural Networks
Let’s see the real-life examples of neural networks and the use of machine learning in healthcare. It will also be fair to consider the major limitations and difficulties of using neural network technologies in real clinical practice, which prevent the development and distribution of the technology.
Neural Network and Genetics
DNA analysis is a promising and actively developing area for applying neural networks. For example, a tool developed by Michigan State University carries out genetic research and allows scientists to determine the height of a person through their genome to within three centimeters, predict the development of serious diseases such as cancer, stroke, and heart attack, identify mutations that affect bone density, and even predict the level of education that a person can achieve.
Medicine Development
The first drug created with artificial intelligence to enter clinical trials was the drug DSP-1181. DSP-1181 was developed by Exscientia in conjunction with a Japanese pharmaceutical company.
DSP-1181 is a 5-HT1A serotonin receptor agonist, and it is indicated for the treatment of patients with obsessive-compulsive disorder (OCD). Typically, it takes up to five years to accomplish this kind of research and develop this kind of medicine (the drug discovery stage). Artificial intelligence completed this task in just a year.
The first phase of clinical trials of the drug were scheduled for March 2020. There is no information yet on whether these plans were affected by the COVID-19 pandemic.
Speech Recognition
Physician workflows involve more than patient consultation or research. A significant part of the time is spent on filling out various kinds of documentation. Neural network technologies can also help the doctor with this routine work.
For instance, Voice2Med saves physician time by voice-filling medical records. The service includes specialized dictionaries, which allow them to recognize medical terms correctly.
Diagnosis
Neural networks are non-linear systems that allow for much better data classification than commonly used linear methods. When applied to medical diagnostics, neural networks make it possible to increase the method's specificity without reducing its sensitivity significantly.
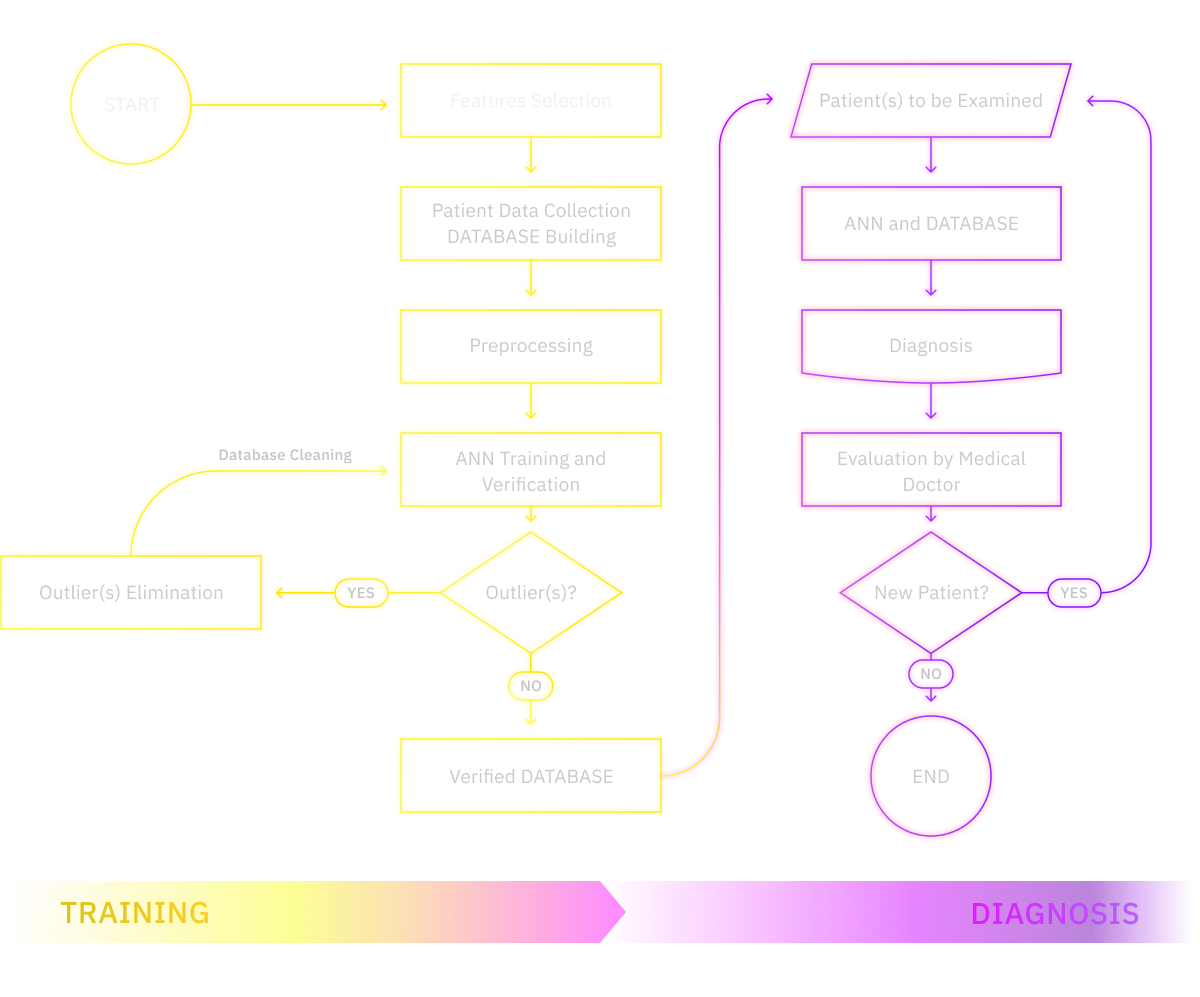
Note, the neural network that diagnoses a heart attack used to work with a large set of parameters, which affects diagnoses in an unclear way. Nevertheless, neural networks were able to find hidden patterns in multidimensional data, and were able to make smart decisions.
“What makes using neural networks special? They are not programmed and do not use any inference rules for making a diagnosis. Instead, they learn to do this by observing several practical examples and cases. Well-trained artificial neural networks can process various kinds of medical data and enhance the way health professionals diagnose even the most complicated issues”
— Vlad Medvedovsky CEO at Proxet, a custom software development solutions company.
It's worth mentioning that neural networks differ from expert systems, the development of which in the 70s took place after the temporary "victory" of Artificial Intelligence over the approach to modeling memory with pattern recognition and generalization, which was based on studies of the neural organization of the brain.
Diagnostics is a special case of event classification, and the most valuable thing here is the classification of those events that are not represented in the training neural network set. Here the advantage of neural network technologies is manifested loud and clear: they can carry out such a classification, generalizing previous experience and applying it in new cases.
Limitations and Issues
The first obstacle is developing a medical neural network. You’ll need a lot of data to train artificial intelligence in the right way. For analysis of medical images, data is pictures with markings on objects.
There are publicly available datasets, but most of them are only available for non-commercial use. Besides, the markup can be different, so it is not always suitable for a specific task.
This is why developers cannot do without collecting datasets. It is all about training your own neural network model. This, in turn, requires doctors' direct involvement, in both collecting and marking up data as well as at other development stages. Without feedback, the product will be "detached" from real clinical practice and will not be able to sufficiently take into account the specifics of the physicians' work.
Another obstacle is related to introducing a final product into the work processes of medical institutions. And the major issue here is the lack of specific standards stated in the legislation that governs the use of such technologies in medicine.
Neural Networks Architecture for Healthcare
New types of neural network architectures are emerging all the time, and you can get confused about them. Most systems related to healthcare are based on so-called “gold standard” architectures. The major point here is the applicability of the particular neural network to your special case, i.e. your project.
Of course, there are different types of neural networks, and you have to be an industry expert to understand all the nuances. If you aren’t, you’ll need a decent consultation.
What’s more, many people get confused when it comes to big data analytics architecture. That is yet another argument in favor of seeking a specialist’s opinion.
The Relevant Examples in Healthcare
The old-school example of a diagnostic program is the cardiology package developed by RES Informatica and the Center for Cardiac Research in Milan. The program allows for non-invasive cardiac diagnostics based on the recognition of tachogram spectra. A tachogram is a histogram of the intervals between successive heartbeats. Its spectrum reflects the balance of the activities of the sympathetic and parasympathetic nervous system of a person, which changes specifically for various diseases.
Perhaps the largest and most talked-about project of using AI in healthcare is Watson, IBM’s cognitive system. Initially, this solution was trained and then applied in oncology. IBM Watson performed spectacularly, making an accurate diagnosis and suggesting an effective cure for each patient.
More than 30 billion medical images were analyzed to train IBM Watson. As part of building Watson’s medical imaging capability, IBM bought Merge Healthcare—a leading provider of medical image handling and processing, interoperability, and clinical systems—for $1 billion. IBM also added 50 million anonymous electronic health records, which IBM acquired through its purchase of Explorys, a startup. There are a lot of examples of neural network implementation in healthcare. After all, everyone is investing billions of dollars and hunting for great solutions.
Several Simple Steps to Start Using Neural Networks
So, still wonder where to start?
- First, make up your mind. You have to be sure you need it. Implementing such a complicated system is not a cakewalk. Without resolve, you’ll be “drowning” in your hesitations again and again.
- Second, take a look at modern ready solutions. The leaders of the market always have something to offer. Examine all the offers carefully, and try to note the most suitable options.
- Third, consider developing a “personal solution” or in-house solution. But you should remember that this is a costly process that takes a lot of time. Therefore, you have to know for sure what you want at this point.
- Fourth, be ready for changes. Open-minded leaders make the majority of mistakes, but also usually end up winners.
- Fifth, make the first step. Come up with a coherent strategy, sober mind, and brave heart.
Talking about the market's professionals and leaders, it would be fair to mention the Proxet team and its solutions. Proxet is where complex problems in the fast-evolving healthcare and pharma sectors are solved. Check out this medical research portal, and see for yourself. Furthermore, Proxet are experts in startup partnerships and healthcare digital transformation.