The benefits of medical imaging cannot be overstated. Doctors, provided with the ability to “look inside” patients, can diagnose diseases more accurately, devise better treatments, and save countless lives.
As healthcare technology advances and healthcare serves more people, the demand for medical imaging surges. At the same time, the supply of skillful and knowledgeable medical imaging specialists tightens. COVID-19 puts even more strain on the industry. IMV Medical Information Division, a specialized marketing research company, reported that 6.5 million MRI scans had been postponed in the USA alone due to the pandemic. This backlog will be hard to cope with.
As such, making medical imaging more efficient is more urgent than ever.
However, doctors have already found a powerful ally in Artificial Intelligence. A healthcare professional can now rely on an algorithm images can be processed with. The algorithm can help to get and analyze picture faster, improving outcomes and convenience for patients and doctors. In this article, we are going to describe how exactly AI can help with medical image analysis.
Data Acquisition and Image Reconstruction
Medical imaging is a complex process, requiring expensive, intricate equipment and highly-skilled professionals to be of real value. A new generation of tools based on artificial intelligence can have a positive and significant impact on this field. Healthcare professionals get several benefits from medical imaging with artificial intelligence:
- Increasing throughput of medical scanners. AI tools make imaging faster. Medical images are complex entities; composites of several different “shots.” New ML-based software for Positron Emission Tomography (PET) scans reduces the number of counts required to reconstruct an image. As a result, the amount of radioactive tracer and time spent at each bed position can also be reduced. Reduced doses of tracer and time in scans helps with imaging pediatric patients and those requiring long-term surveillance.
- Improving patients’ comfort. Shorter imaging sessions means enhanced efficiency as well as a better patient experience. For instance, MRI studies may be stressful, and up to 30% of patients suffer frank anxiety reactions. The latest ML-enhanced image space-based IR package offers up to 30% shorter scan time. As a result, a patient can get, for example, a brain scan picture as fast as possible.
Besides, AI can have more patient-focused benefits for MR imaging. Several companies are working on tools to eliminate distortions caused by motion. In the future, scans won’t have to be repeated even if the patient inadvertently moves during the procedure.
- Enhancing patients’ safety. Over the last decade of computer tomography, so-called iterative reconstruction (IR) has replaced the filtered back projection (FBP) image reconstruction techniques. The latter requires lower X-ray dosing but produces pictures, requiring a different approach to analysis. ML models, trained on traditional CTs, allow both to use an IR and offer interpreting physicians more familiar images. As a result, patients are exposed to less X-rays, and doctors can read the scans more easily.
“As we emerge from the COVID-19 crisis and the volume of imaging deferred during social distancing inevitably returns, additional benefits will come into play as more efficient image acquisition will leave more time to devote to the safe transition of exam rooms between patients.”
— Lawrence Tanenbaum, MD, Vice President and Medical Director Eastern Region, RadNet Inc
Biomedical Signal and Image Processing with AI
The human organism is a complex system generating electrical, thermal, mechanical, and many other signals that are called biomedical. Measuring skin temperature or conductance, making an electrocardiogram (ECG), or electromyography (EMG, assessing the health of muscles and the nerve cells controlling them) are examples of recording the dynamic changes in the human body.
The automatic analysis of biomedical signals has become a key part of identifying symptoms of diseases and providing meaningful information for different applications in physiology and sports medicine. The rise in the popularity of smart wearables in recent years has made this data more abundant.
Scientists believe that recent advances in Artificial Intelligence can help in managing and analyzing large biomedical datasets. Applying Artificial Intelligence to datasets may lead to better clinical decisions and real-time applications. As we’ve already mentioned, AI has been successfully applied to medical imaging, and biomedical signal processing can also benefit from this technology.
Biomedical signal and image processing involves analysis, classification, and manipulation of those signals by various research methods: filtering, feature extraction, spectral analysis, etc. Innovative computational techniques involving both signal and image processing reveal new solutions to the existing challenges.
“Novel signal processing methods have assisted in revealing information that entirely altered the previous approaches taken in the diagnosis of different diseases. To analyze biomedical signals, biomedical engineers use different types of signal processing and machine learning techniques. By using intelligent biomedical analysis tools, the signals can be analyzed by software to help physicians gain greater insights and to make better decisions in clinical assessments.”
— Professor Abdulhamit Subasi, Effat University, Jeddah, Saudi Arabia
The recent studies of AI-based biomedical circuits and systems include:
- The use of ECG data and artificial neural network for cardiac arrhythmia detection and classification;
- EEG (electroencephalogram) and neural signal processing with the help of machine learning to localize epileptic focus;
- EMG-based hand gesture classification systems utilizing deep learning image processing.
However, the challenge lies deeper than improving AI algorithms. Researchers recommend that both the algorithm and the hardware should be optimized to meet performance, accuracy, and power consumption requirements. That will make systems for biomedical signal analysis more practical and efficient.
Advanced Tools: Machine Learning and Deep Learning in Medical Image Analysis
AI-based medical imaging is used in many healthcare segments. Here is a brief overview of how it is utilized for various purposes:
- Cardiovascular Imaging. A comprehensive cardiac examination can be done with highly integrated software and the required MRI tools. With imaging, invasive diagnostic procedures, such as examining surgery, angiography, and cardiac catheterization, can be avoided.
“There are a lot of examples of how AI is making cardiovascular disease diagnoses faster and more accurate. Custom software development solutions, using machine learning, can automate even a complex cardiac analysis. Advanced MRI techniques outpace conventional imaging, delivering high-quality images.”
— Vlad Medvedovsky CEO at Proxet
- Breast Imaging. Novel imaging techniques such as the Digital Breast Tomosynthesis (DBT) or 3-D mammography improve lesion visibility and allow doctors to conduct tissue analysis in greater detail. Together with ultrasound and MRI, this technology boosted the accuracy of clinical diagnosis. DBT can even detect breast cancer and potential abnormalities more quickly and precisely. AI also helps radiologists to categorize patients based on individual medical history and breast density. Thus, the right interpreting radiologists can be chosen, and the workflow can be streamlined.
- Lung Imaging. Doctors use Machine Learning image analysis to study lung parenchyma, vasculature, and airways. Technology improves efficiency in lung cancer screening, providing a fast and high-resolution assessment. As a result, lung cancer lesions can be detected successfully. For instance, Infervision, a Chinese artificial intelligence start-up, developed an augmented CT screening solution (AI—CT) that can accurately spot suspicious lesions in CT scans. This technology has facilitated the treatment of lung cancer.
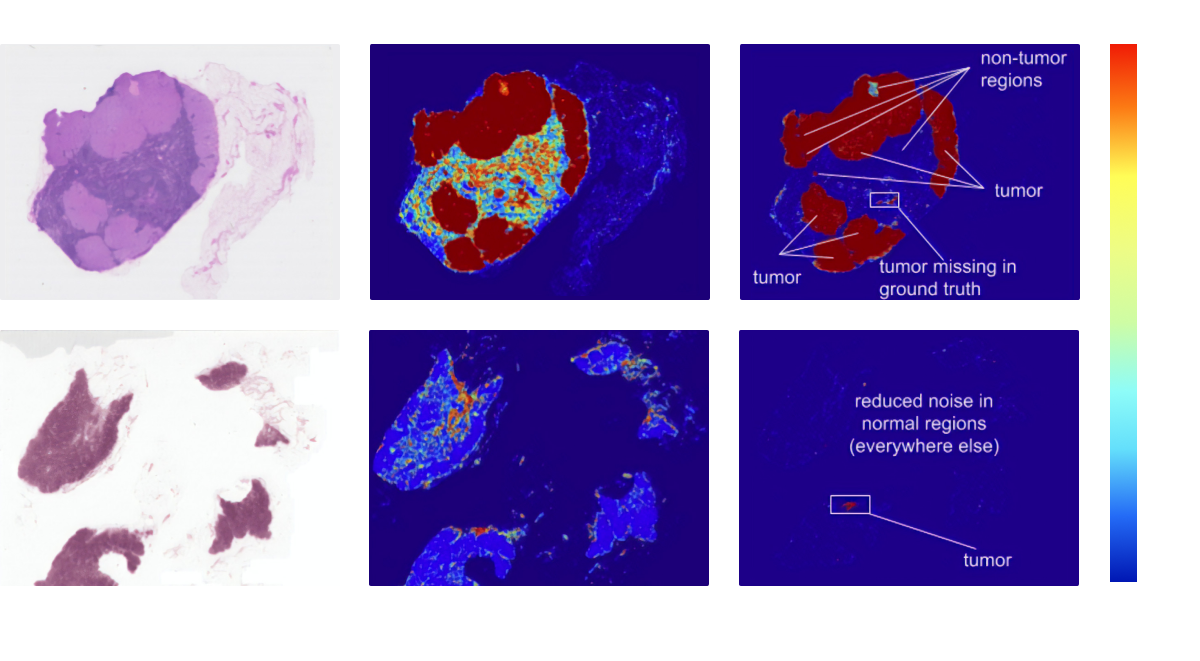
- Neurological Imaging. AI has become an important instrument in the diagnosis of brain injury, brain trauma, and certain diseases. In combination with computerized tomography (CT) or computerized axial tomography (CAT) and magnetic resonance imaging (MRI), this technology allows physicians to obtain a comprehensive map of the central nervous system.
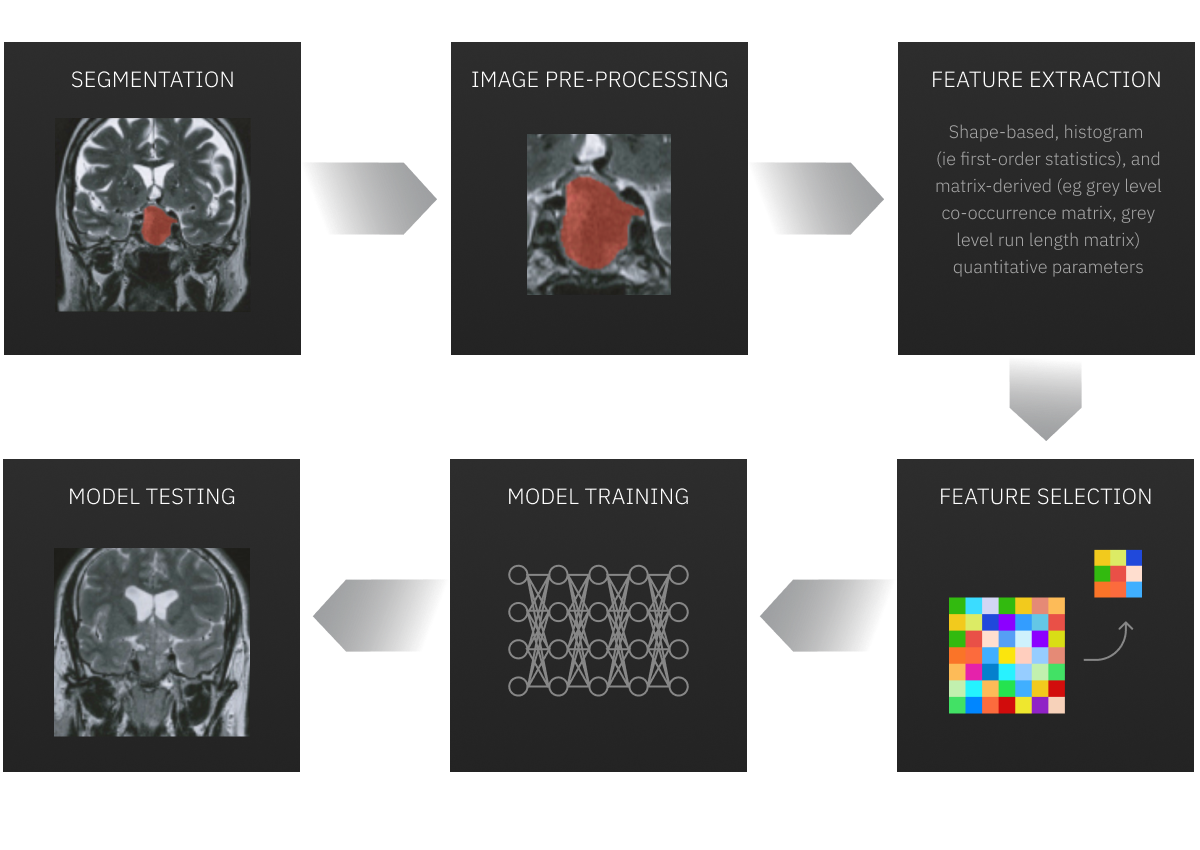
AI-based Tools to Start Analyze Medical Images
The market for medical image analysis software is booming. Grand View Research estimated its size at $2.6 billion and predicted that by 2027 it would reach $4.5 billion. The implementation of AI technologies will probably be one of the factors to boost its growth shortly.
Some of the recent examples of AI-based software include:
- QuantX, computer-aided diagnosis software, using MRIs to help radiologists in the assessment and characterization of breast abnormalities;
- ProFound AI, analyzing mammograms for suspicious lesions. The study indicates this software improves detection rates by 8%;
- IDx-DR, a computer-aided diagnosis program that scans eye images for signs of diabetic retinopathy;
- OsteoDetect, software for Х-ray image processing that, with the help of machine learning, detects and highlights distal radius fractures.
There are also some open source solutions to build your system. For example, MedPy is a free library for medical image analysis with Python, offering basic functionality for working with large images of many dimensions.
However, to build complex software for medical image analysis custom-tailored to your needs, you will likely need help from a team of professionals. Proxet has worked with many clients in the healthcare industry and equipped their businesses with AI-based solutions, for example, triage chatbots. Our team has profound machine learning expertise, which includes image recognition. We will do our best to provide you with the most suitable software solution as fast as we can.