Did you know that without clinical trials, there wouldn’t be vaccines for COVID?
In fact, the progress of medicine very much depends on clinical research and, in turn, clinical trials. It’s a process that helps medical professionals explore and evaluate the effectiveness of new treatments, a process whose significance was highlighted after the recent COVID pandemic.
With that in mind, we should not be surprised that it is also a growing market in the medical sector. According to market research conducted by
Mordor Intelligence, the clinical trial market was valued at around $39 billion back in 2021, and it is expected to grow to nearly $53 billion by 2027, with a compound annual growth rate (CAGR) of 5.05 percent.
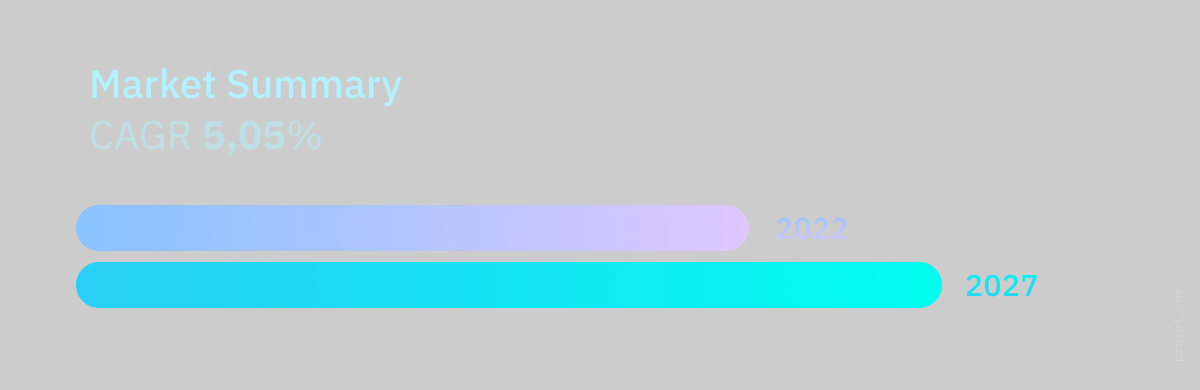
To put it simply, as long as people get sick—and we certainly will, at some point in our lives—we will need to find a way to prevent and cure our diseases. In order to do so, we will need to conduct medical research and clinical trials to discover the effectiveness of new cures.
And as the aging population begins to constitute the majority of the world society, along with it also comes the prevalence of chronic illnesses—according to research, the incidence of chronic illnesses rises dramatically with age. In the United States, about 80 percent of adults above the age of 65 suffer from one chronic illness, with 50 percent suffering from two or more.
But how does artificial intelligence (AI) come into the picture? What exactly are AI clinical trials? What do machine-learning studies have anything to do with clinical research and trials?
That’d be the focus of today’s article. Buckle up as we discover how AI can help cure cancer (sort of).
What are Clinical Trials?
Before we go into the use of machine learning in clinical trials, we need to understand what clinical trials are first and what exactly the process entails.
In simple terms, clinical research can be seen as medical research that involves people. Meanwhile, clinical trials are a type of clinical research that’s focused on exploring and evaluating the outcome of medical, surgical, or behavioral interventions.
Clinical trials are the primary method for researchers to discover whether a new treatment, be it a new drug or medical device, is safe and effective for people. Oftentimes, the results are also compared to existing/standard treatments to evaluate their effectiveness—an aspect where AI can be used, which we will come back to later.
Types of Clinical Trials
According to the US Food & Drug Administration (FDA), clinical research can also be divided into different groups and categories based on the nature of the research itself.
- Treatment Research—exploring the use of medication, psychotherapy, new devices, or new approaches to surgery or radiation therapy.
- Prevention Research—exploring ways to prevent disorders from developing or returning. Studies can include medicines, vitamins, vaccines, minerals, or lifestyle changes.
- Diagnostic Research—discovering ways to identify a particular disorder or condition.
- Screening Research—exploring ways to detect certain disorders or health conditions.
- Quality of Life Research—exploring ways to improve the quality of life for individuals with a chronic illness.
- Genetic studies—finding ways to improve the prediction of disorders by identifying and understanding the roles of genetics in illnesses. Exploring the likelihood of how certain genes play a role in certain illnesses can help develop tailor-made treatments based on a patient’s genetic makeup.
- Epidemiological studies—identifying the patterns, causes, and control of disorders in groups of people.
Clinical trials can also be separated into two categories—“outpatient” trials refer to those where the patients do not need to stay overnight, while “inpatient” refers to those where the patients will need to spend a night or more in the medical institutions.
Phases of Clinical Trials
Again to the same article by the FDA, clinical trials can also be divided into four phases, providing different answers to different questions.
Phase I trials
- Testing an experimental drug or treatment in a small group of people for the first time
- Evaluate the treatment’s safety, determine a safe dosage range, and identify side effects.
Phase II trials
- Providing the experimental drug or treatment to a larger group
- Further evaluation of its effectiveness and its safety.
Phase III trials
- Provide the experimental study drug or treatment to large groups of people
- Confirm its effectiveness, monitor its side effects, and compare it to commonly used treatments
- Gather information that will allow the experimental drug or treatment to be used safely
Phase IV trials
- Post-marketing studies conducted after a treatment is approved for use by the FDA
- Provide additional information, including the treatment or drug’s risks, benefits, and best use.
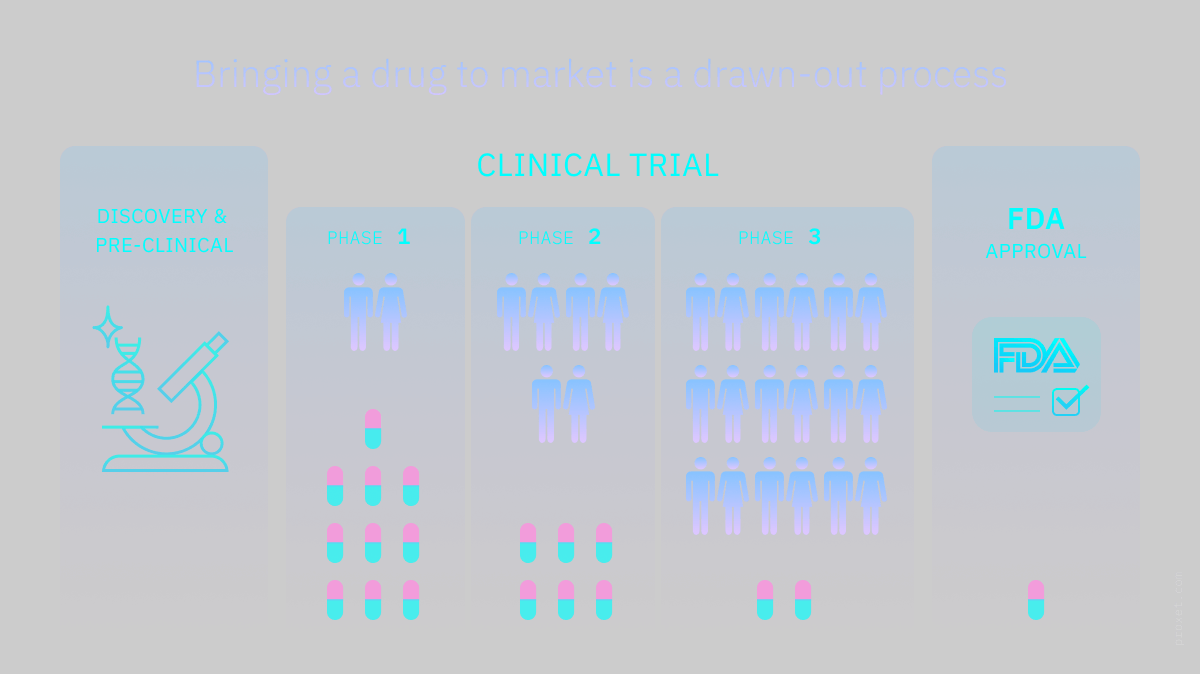
Challenges of Clinical Trials
Despite the increased funding, the productivity of the biopharmaceutical industry—an industry largely reliant on clinical trials—has remained relatively stagnant.
"Everyone is affected by the risk of a drug failing in its clinical trial process.— Andrew Lo, director of the MIT Laboratory for Financial Engineering.
According to an article by Clinical Reader, new molecule entity (NME) and biologic license application (BLA) approvals have seen few increases in recent years despite the rise in funding and developments. So why’s that the case?
Patient Matching
Clinical trials are a slow and costly process, and that cost stems from a myriad of factors. One of them is finding the right patient for the trials. The lack of matching patients can also translate to a small sample size, which renders effective analysis extremely difficult.
Lack of Data
Similar to the challenges in finding the right patients for the trials (and having them participate in the trials), the lack of data is another challenge numerous clinical trials face. During the later stages of clinical trials, more patients and, along with that, more data are required to evaluate the outcome and performance of the experimental treatments effectively.
Limited Clinical Trial Analytics
As a lot of clinical trials can be bound by geographical boundaries, obtaining data from different sample groups across the globe can be challenging. Thus the challenges in clinical trial analytics arise.
Loss of Structured Data
One of the peculiar challenges in clinical trials is the loss of valuable structured data due to the old-fashioned methods employed by some institutions. For example, medical methods are often faxed and then converted back into PDF files for storage, thus negating all the benefits that structured medical records had to offer, ultimately becoming a hindrance in clinical trials data analytics.
These are only some of the challenges medical researchers encounter while conducting clinical trials—but these are also some issues that can be overcome by AI and machine learning.
Artificial Intelligence Clinical Trials and Clinical Trial Analytics
While AI is more of a general concept compared to machine learning, which refers to the algorithms used to simulate human logic, we are not going to dig deep into the semantics here.
A lot of the challenges we mentioned above can be aided, or even resolved, by the use of machine learning. ML, in medical terms, can be seen as the process of automation while handling a huge amount of data with minimal human intervention, greatly improving the effectiveness and accuracy of the process. On top of that, it can also identify the correlations between different data that can otherwise go unnoticed by ordinary analysis.
In recent years, numerous companies providing software development services have already tapped into the market to explore the use of AI and machine learning in clinical trials.
"We have worked with different medical institutions over the years, and they have all been showing promising results by integrating AI in their approach."
— Vlad Medvedovsky, CEO at Proxet (ex - Rails Reactor) - a custom software development company.
Data Mining
One thing that machine learning excels at is data mining, and this is a technology that can be utilized in clinical research and trials. As we mentioned above, finding patients who match the requirements for clinical trials can be a tedious process, but pharmaceutical machine learning can help speed up the process by going through existing medical records to find matching patients.
By using structured records, researchers are able to retrieve lists of patients who match the criteria for an upcoming clinical trial, greatly reducing the time to within minutes. After identifying the right patients, requests can then be sent for their consent to participate in the trials. In fact, said workflow might have been patented in the EU already.
On top of that, some research has explored the use of machine learning in navigating through the massive amount of data generated by pharmaceutical clinical trials and helping researchers identify meaningful data in clinical trial analytics.
Natural Language Processing (NLP)
One of the emerging fields in machine learning is Natural language processing (NLP), and it can also be used in clinical trials. A huge amount of data and reports are normally involved in pharmaceutical trials, many of which contain unstructured data where entering them manually would be next to impossible.
With the use of NLP, however, computers can extract meaningful data from reports and further incorporate them into the research process. It’s not just about finding keywords either—NLP can look at the medical records and identify conditions that might not be specified by the doctor initially, in turn further enlarging the pool of possible patients and accurately evaluating the patients’ conditions after the trials.
AI Forecast
Clinical trials are a serious undertaking, and utmost care must be taken before starting the trials. Not only that, but attempts at projecting the outcome of the trials before they start are also a way to determine whether it’s worth proceeding. This is where artificial intelligence clinical trials found their use.
Among the numerous research done on this topic, one of them truly stood out—researchers at the Leiden and Rotterdam medical universities conducted
target="_blank" aria-label="undefined (opens in a new tab)" rel="noreferrer noopener">research using Euretos AI Platform data to train a machine learning model for predicting whether a drug would succeed in a clinical trial. The result? A 92.2 percent of accuracy.
ML Models for Clinical Research
Depending on the purposes of the research, different ML algorithms can be used to help facilitate the process and identify the outcomes.
"Leveraging healthcare data sets, ML tools can help assess and optimize compound positioning as well as optimize primary and secondary endpoints in study design.— Lucas Glass, Vice President of the IQVIA Analytics Center of Excellence (ACOE).
K-Nearest Neighbor (K-NN)
K-nearest neighbors (K-NNs) are one of the models used in medical data mining (or any sort of data mining, for that matter). Instead of using the traditional logical regression, K-NNs take a step back and look at how data relate to each other in terms of their Euclidean distance.
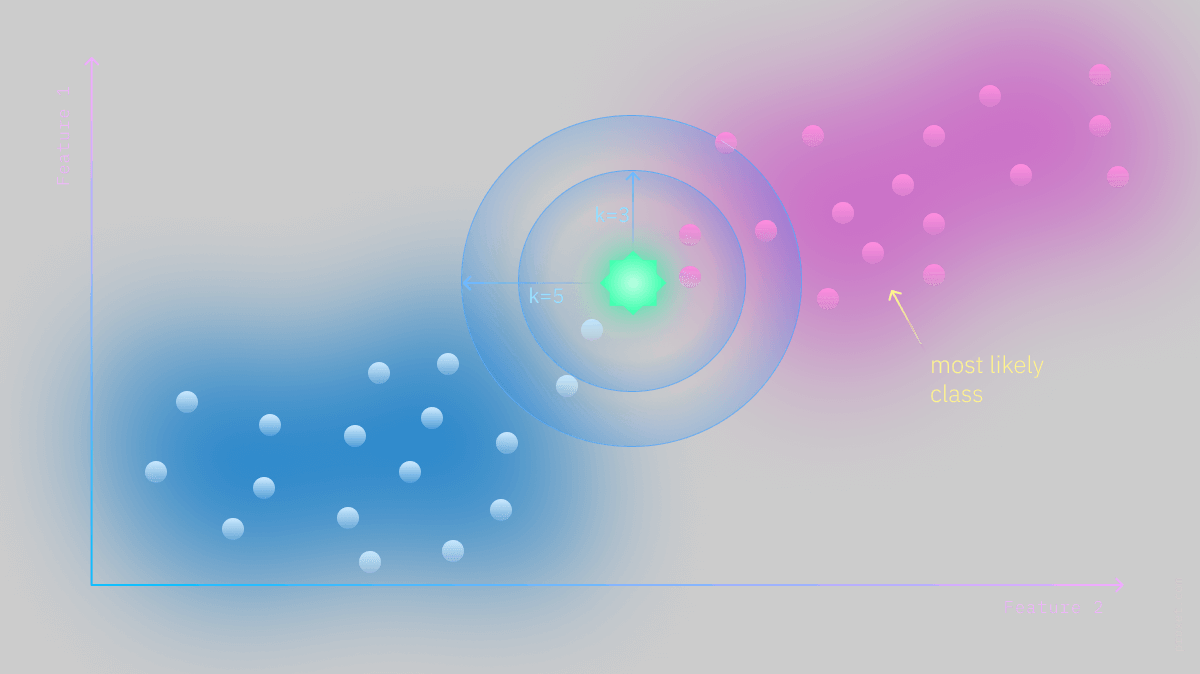
Decision Tree
In certain cases, machine learning decision trees can also be used to classify the outcomes of clinical trials, where the data can then be analyzed further to evaluate the effectiveness of the experimental treatment. In one research, researchers utilized different forms of decision trees (ID3, C4.5, and CART) to classify the outcomes of tuberculosis treatments.
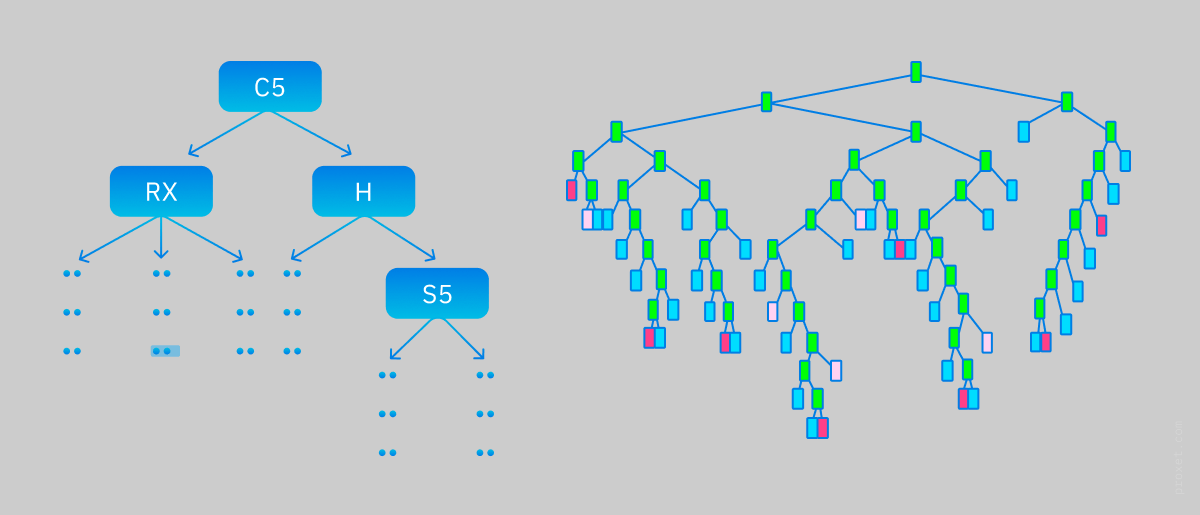
Multiple Regression
Certain machine learning algorithms can help isolate and identify the cause and effect between different variables, which can be very useful in discovering the contributing factors during clinical trials. By using multiple regression, researchers can understand which variables are influencing the observed effects.
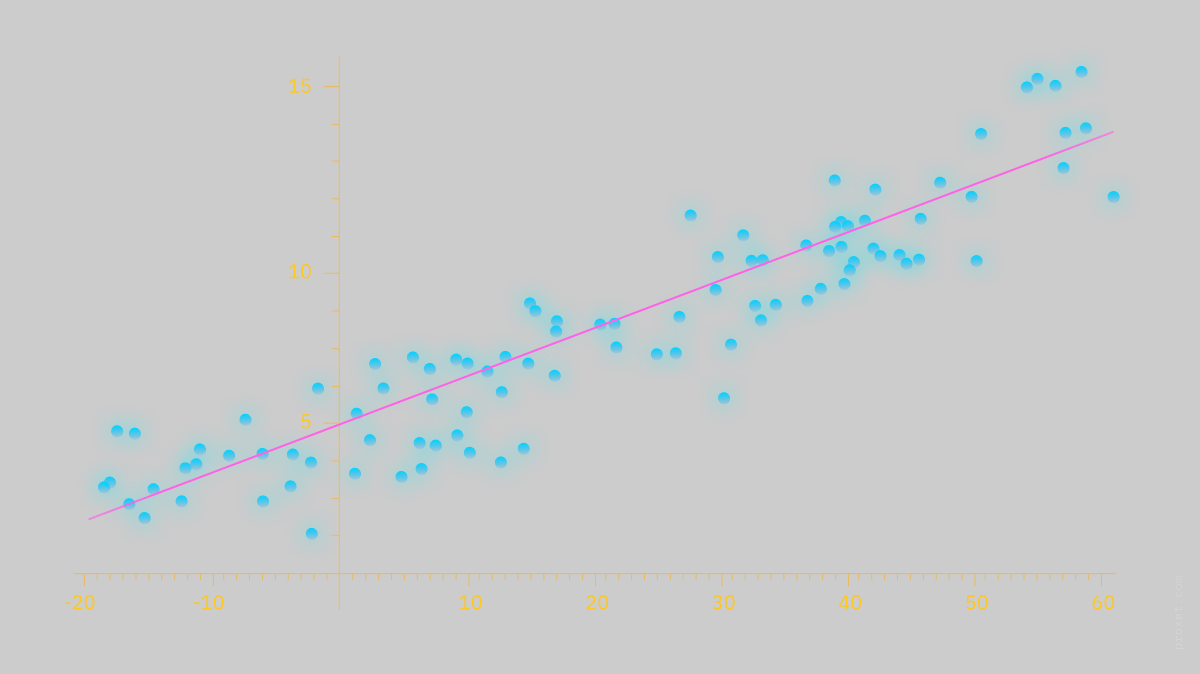
Here are just a few examples of ML models used in clinical trials data analytics. If you’re interested in how these can algorithms can be used in clinical trials, feel free to check out the links embedded throughout this article.
Future of Clinical Research
We merely touched on the surface of how AI and machine learning can shape the future of clinical research and, along with that, clinical trials. Looking at the current development, we have a strong belief that AI will dominate clinical research within the next decade.
From better patient enrollment to automated patient monitoring, AI can change the approach to clinical trials altogether.
Proxet is a software development firm with years of experience in creating AI-based solutions for medical institutions, specializing in both data engineering and NLP to make the best use of available data. Should you be looking for assistance in transforming your medical solutions through the use of AI, don’t hesitate to contact us today.